Being able to analyze information is crucial for solving complicated problems logically. This post will explore why analytical skills are so important in the workplace and includes 50 interview questions about analytical skills.
Get more interview questions.
Sign up for Huntr to access interview questions tailored to the job you're applying for.
What are analytical skills?
Analytical skills are a set of capabilities that allow an individual to solve complex problems by making decisions in a logical, systematic way. These skills involve breaking down large problems into smaller, more manageable parts, identifying patterns and relationships, evaluating information critically, and utilizing logical reasoning to come up with effective solutions. People with strong analytical skills are often able to quickly understand new information, see various perspectives, and make well-informed decisions. These skills are highly valued in many professions, including business, technology, science, and engineering, as they enable individuals to tackle challenges creatively and efficiently.
Why are analytical skills important in the workplace?
1. Enhanced Problem-Solving Abilities
Analytical skills are crucial in the workplace because they empower employees to dissect complex problems, identify patterns, and derive actionable insights. This ability to break down intricate issues into manageable parts and solve them efficiently is invaluable across various scenarios, from daily operational challenges to strategic decision-making.
2. Data-Driven Decision Making
In today’s data-centric world, having strong analytical skills allows individuals to interpret and leverage data effectively. This competency enables employees to make informed decisions based on factual evidence rather than intuition or guesswork. By understanding and applying data analytics, businesses can improve their strategies, optimize processes, and ultimately achieve better outcomes.
3. Improved Communication and Presentation
Analytical skills are not just about crunching numbers or interpreting data; they also enhance one’s ability to communicate complex information clearly and persuasively. Individuals with these skills can translate intricate data findings into understandable, actionable insights for diverse audiences. This ability is essential for convincing stakeholders, informing team decisions, and presenting strategies that are backed by solid analysis.
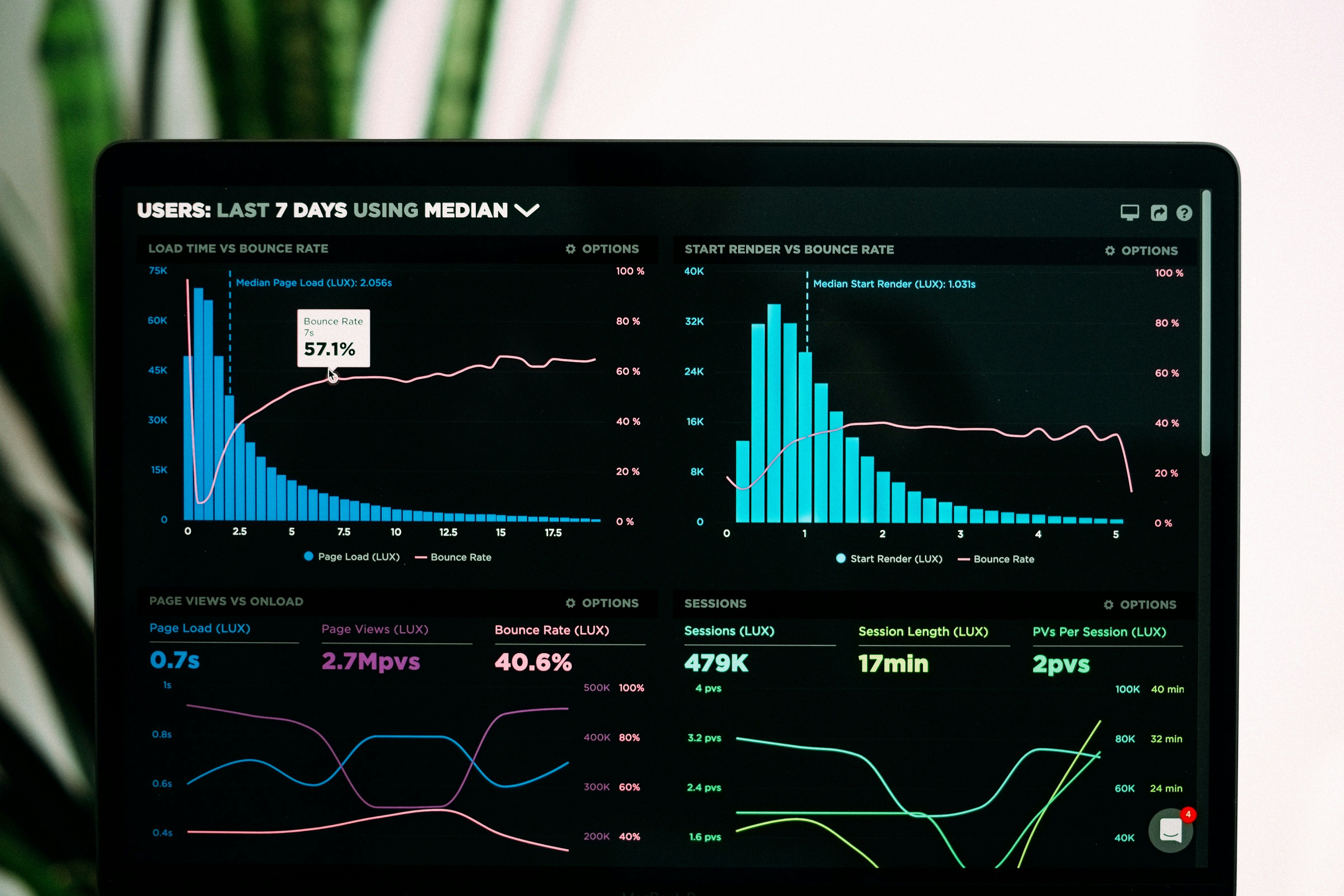
5 Tips for Answering Analytical Skills Interview Questions
When it comes to job interviews, showcasing your analytical skills can set you apart from the competition. Analytical skills refer to your ability to collect and analyze information, solve problems, and make decisions. Whether you're applying for a role in data science, finance, marketing, or any field that requires a keen analytical mind, here are five tips to effectively demonstrate your analytical prowess during an interview:
1. Understand the Question Completely
Before diving into your answer, make sure you fully understand the question. Interviewers often assess analytical skills through complex scenarios or problems. If anything is unclear, don’t hesitate to ask for clarification. Showing that you're ensuring you have all the necessary information before proceeding is part of your analytical process.
2. Describe Your Thought Process
When answering, walk the interviewer through your thought process. Don't just jump to the conclusion. Explain how you gather information, identify key factors, and consider various solutions. This demonstrates your systematic approach to problem-solving and decision-making, which is at the heart of strong analytical skills.
3. Use Real-Life Examples
The best way to prove your analytical abilities is by sharing specific examples from your past experiences. Describe a situation where you faced a challenging problem, how you analyzed the situation, the steps you took to resolve it, and the outcome. Quantify your success with data and results if possible, as this adds credibility to your story.
4. Highlight Tools and Techniques
If you've used any tools, software, or methodologies to aid your analytical processes, mention these in your answers. Whether it's statistical software, a particular framework for decision-making, or specific techniques for data analysis, showcasing your familiarity with these tools demonstrates your practical skills and knowledge in applying your analytical abilities.
5. Showcase Your Soft Skills
Analytical skills are not just about crunching numbers or logical reasoning; they also involve creativity, critical thinking, and the ability to communicate complex information clearly and concisely. Highlight instances where you've had to present your findings to non-technical stakeholders or how you've used your analytical skills to lead a team towards a data-driven decision. This shows that your analytical skills are well-rounded and adaptable to various scenarios.
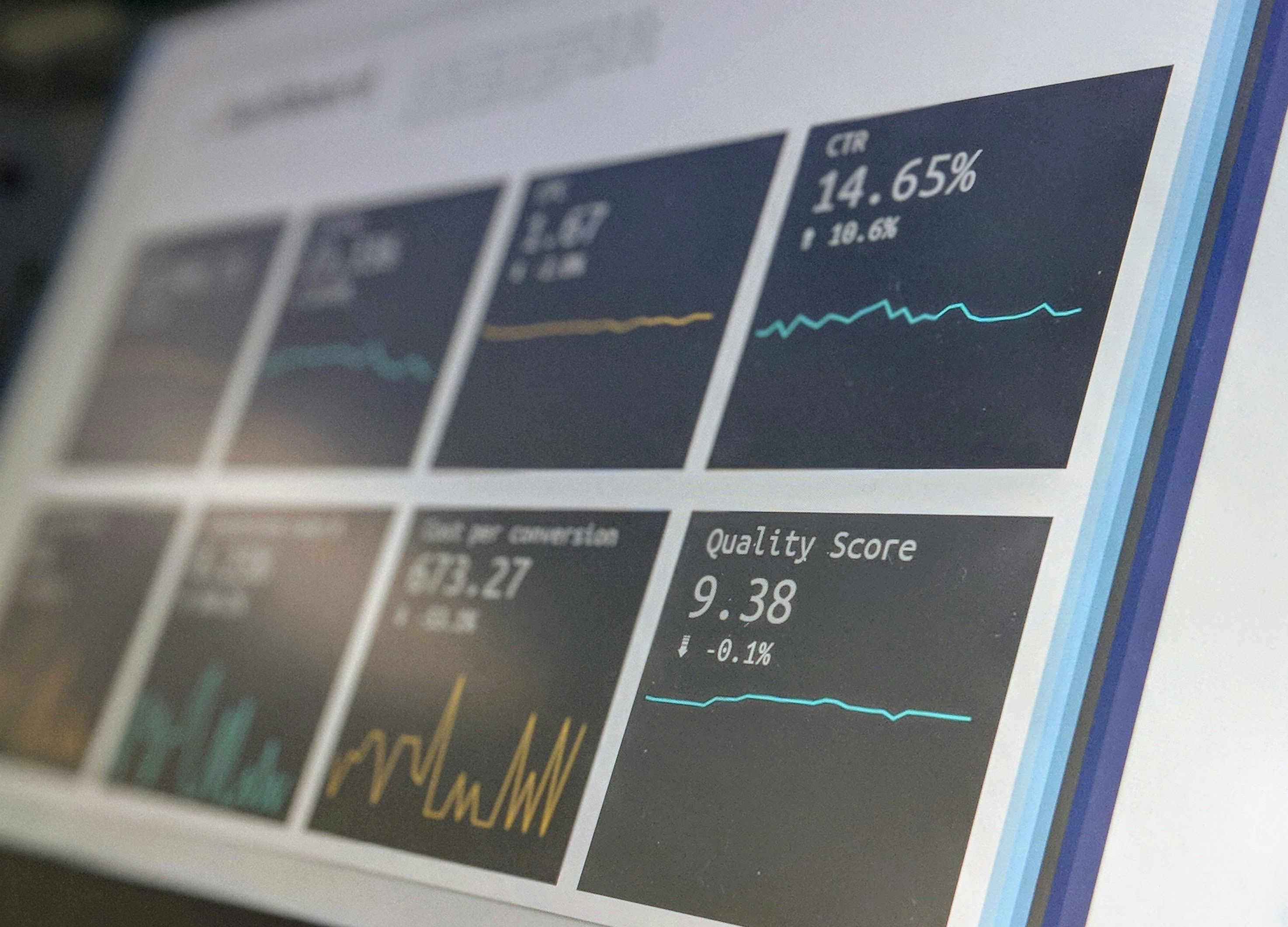
50 Interview Questions About Analytical Skills
1. Can you describe a complex problem you solved using your analytical skills?
Certainly. In my previous role, I was tasked with optimizing inventory management for a retail company facing supply chain disruptions. I conducted a thorough analysis of historical data, supplier lead times, demand patterns, and production capacities. Using statistical models and forecasting techniques, I identified key bottlenecks and developed a dynamic inventory replenishment strategy. This resulted in a 20% reduction in stockouts, a 15% decrease in excess inventory costs, and improved customer satisfaction due to more reliable product availability.
2. How do you approach making decisions that require a high level of analytical thinking?
When faced with decisions requiring analytical thinking, I follow a structured approach. First, I define the problem clearly, breaking it down into manageable components. Then, I gather relevant data from diverse sources, ensuring its accuracy and completeness. Next, I analyze the data using quantitative and qualitative methods, considering various scenarios and potential outcomes. I consult with stakeholders to gain insights and perspectives, and I weigh the risks and benefits of each option before making an informed decision based on evidence and logic.
3. What tools or methods do you use to improve your analytical skills?
I regularly use tools like Excel for data analysis, statistical software such as R or Python for advanced modeling, and data visualization tools like Tableau for presenting insights effectively. I also engage in continuous learning by taking online courses, attending workshops, and reading industry publications to stay updated on the latest analytical techniques and best practices. Additionally, I actively seek feedback from peers and mentors to refine my analytical approaches and enhance my problem-solving abilities.
4. Can you give an example of a time when your analytical skills led to a significant improvement in a project or process?
Certainly. In a recent project, my analysis of customer feedback data revealed a recurring issue with product usability. I conducted usability tests, analyzed user interactions, and identified key pain points. Based on these insights, I collaborated with the design team to implement interface enhancements and streamline user workflows. As a result, user satisfaction scores increased by 25%, and customer complaints related to usability decreased by 30%, leading to a more positive user experience and higher product adoption rates.
5. How do you ensure your analytical conclusions are accurate and reliable?
To ensure accuracy and reliability in my analytical conclusions, I employ several validation techniques. First, I verify the quality and integrity of the data, checking for inconsistencies, outliers, and missing values. I cross-validate my analyses using different methods or models to confirm consistency and robustness. I also conduct sensitivity analyses to assess the impact of assumptions or uncertainties on the results. Additionally, I seek peer review and feedback from subject matter experts to validate my findings and address any potential biases or errors.
6. What steps do you take when your analysis leads to unexpected or counterintuitive results?
When faced with unexpected or counterintuitive results, I take a systematic approach to investigate further. I review the data collection process, checking for anomalies or data entry errors. I reassess my assumptions and methodologies, considering alternative explanations or factors that may have influenced the outcomes. I consult with colleagues or experts to gain different perspectives and brainstorm potential insights or interpretations. I conduct additional analyses or experiments to validate or refute the unexpected findings, ensuring a thorough and rigorous approach to problem-solving.
7. How do you prioritize tasks when multiple issues require your analytical attention?
When multiple issues require analytical attention, I prioritize tasks based on several factors. I assess the urgency and impact of each issue on strategic goals or project timelines. I consider the availability of resources, such as data, expertise, and tools, needed to address each issue effectively. I consult with stakeholders to understand their priorities and expectations. I use techniques like the Eisenhower Matrix to categorize tasks based on importance and urgency, ensuring that critical issues are addressed promptly while maintaining a balance across various analytical initiatives.
8. In what way have you used analytical skills to predict future trends or behaviors in your field?
I've used analytical skills to predict future trends by analyzing historical data, market research, and consumer behavior patterns. For example, in my previous role in marketing, I developed predictive models using machine learning algorithms to forecast customer preferences and purchasing trends. By leveraging data on customer demographics, purchasing history, and online interactions, I identified emerging trends and recommended targeted marketing strategies that led to increased customer engagement and revenue growth.
9. Can you discuss a situation where you had to use both qualitative and quantitative analysis to solve a problem?
Certainly. In a project to improve employee satisfaction, I used a mixed-methods approach combining qualitative surveys and quantitative data analysis. I conducted surveys to gather qualitative feedback on factors influencing employee morale and engagement. Simultaneously, I analyzed quantitative data from employee performance metrics, turnover rates, and feedback scores. By triangulating both types of data, I identified key drivers of satisfaction, such as leadership communication, work-life balance, and professional development opportunities. This holistic approach allowed us to develop targeted interventions that addressed underlying issues and improved overall employee satisfaction levels.
10. How do you balance intuition and analytical reasoning in your decision-making process?
I believe in leveraging both intuition and analytical reasoning in decision-making. I use intuition to generate initial hypotheses, identify patterns, and guide creative problem-solving. However, I balance this with rigorous analytical reasoning by gathering evidence, conducting data analysis, and evaluating the potential outcomes of different options. I integrate insights from both intuition and analysis to make informed decisions that consider both quantitative data and qualitative insights, ensuring a comprehensive and well-rounded approach.
11. Can you explain a scenario where your initial analysis was incorrect, and how you addressed the situation?
In a project involving sales forecasting, my initial analysis underestimated demand due to overlooking seasonal trends. Upon reviewing actual sales data, I realized the oversight and promptly conducted a thorough analysis of historical sales patterns, market dynamics, and external factors. I adjusted the forecasting model to incorporate seasonal adjustments and improve accuracy. I also implemented regular monitoring and recalibration processes to catch and correct such discrepancies proactively, ensuring more accurate future forecasts.
12. How do you stay up-to-date with analytical techniques and tools in your industry?
I stay up-to-date with analytical techniques and tools by actively participating in professional development opportunities such as workshops, webinars, and industry conferences. I also engage in continuous learning through online courses, reading industry publications, and following thought leaders in data analytics. Additionally, I collaborate with colleagues and participate in cross-functional projects to learn from diverse perspectives and exchange best practices in analytical methodologies.
13. What challenges have you faced when conducting analysis, and how did you overcome them?
One challenge I faced was dealing with incomplete or inconsistent data sets that affected the accuracy of my analysis. To overcome this, I developed data cleaning and preprocessing techniques to identify and rectify errors, missing values, and outliers. I also collaborated with data engineers to improve data quality, streamline data integration processes, and ensure data integrity for more reliable analyses.
14. How do you communicate complex analytical findings to non-technical stakeholders?
I communicate complex analytical findings to non-technical stakeholders by using clear and concise language, visualizations, and storytelling techniques. I focus on translating technical concepts into actionable insights and key takeaways that resonate with stakeholders' priorities and objectives. I use data visualization tools like charts, graphs, and dashboards to present information visually and facilitate understanding. I also engage stakeholders in interactive discussions, solicit feedback, and provide contextual explanations to ensure comprehension and alignment with decision-making processes.
15. Can you describe a project where you had to analyze a large set of data? What was your approach?
Certainly. In a project focused on customer segmentation and targeting, I had to analyze a large dataset containing demographic, behavioral, and transactional data for thousands of customers. My approach involved several steps: first, I cleaned and prepared the data, addressing missing values and outliers. Next, I conducted exploratory data analysis to understand patterns and trends within the dataset. I used statistical techniques such as clustering and classification algorithms to segment customers based on their characteristics and purchasing behaviors. Finally, I applied predictive modeling to identify high-value customer segments and develop targeted marketing strategies tailored to each segment's preferences and needs.
16. How do you deal with ambiguity or incomplete information when performing an analysis?
When faced with ambiguity or incomplete information, I adopt a systematic approach to address uncertainties and mitigate risks in my analysis. I begin by clearly defining assumptions and limitations, acknowledging areas of uncertainty or data gaps. I conduct sensitivity analyses and scenario planning to assess the potential impact of varying assumptions or missing data on outcomes. I also seek input from subject matter experts, collaborate with cross-functional teams, and triangulate data from multiple sources to validate findings and ensure robustness in my analysis. Additionally, I document my methodologies, assumptions, and decision-making processes transparently to facilitate discussions and informed decision-making.
17. What role do you believe analytical skills play in team collaboration and problem-solving?
Analytical skills are crucial in team collaboration and problem-solving as they enable data-driven decision-making, evidence-based insights, and objective assessments of problems and opportunities. Analytical skills allow teams to leverage data, analytics tools, and methodologies to diagnose root causes, identify trends, and formulate effective solutions. They promote clarity, transparency, and alignment in communication by providing empirical support for recommendations and facilitating consensus-building among team members. Analytical skills also foster innovation, continuous improvement, and learning within teams, driving performance excellence and competitive advantage.
18. Can you discuss a time when your analytical skills helped you identify a business opportunity?
In a previous role, I used analytical skills to identify a business opportunity by analyzing market trends and customer feedback data. Through sentiment analysis and customer segmentation, I discovered a growing demand for eco-friendly products among a specific demographic segment. Leveraging this insight, I recommended developing a new product line of sustainable alternatives, which resulted in increased sales, expanded market share, and enhanced brand reputation, demonstrating the value of analytical skills in identifying and capitalizing on business opportunities.
19. How do you differentiate between correlation and causation in your analysis?
I differentiate between correlation and causation by applying rigorous analytical methods and considering causal inference frameworks. While correlation indicates a statistical relationship between variables, causation implies a direct cause-and-effect relationship where one variable influences the other. To establish causation, I consider temporal precedence, plausible mechanisms, and experimental design principles such as randomized controlled trials or quasi-experimental designs. I also use statistical techniques like regression analysis, propensity score matching, and causal inference models to control for confounding variables and assess causality more robustly in my analysis.
20. What is your process for validating the data you use in your analysis?
My process for validating data involves several steps: first, I assess data quality by checking for completeness, accuracy, consistency, and relevance. I conduct data cleaning and preprocessing to address missing values, outliers, and data entry errors. Next, I verify data integrity by comparing data from different sources, conducting cross-validation checks, and reconciling discrepancies. I also validate data against known benchmarks or external benchmarks, ensuring alignment with expected patterns or distributions. Additionally, I engage domain experts, conduct data audits, and document data validation procedures to ensure transparency and reliability in my analysis.
21. How have your analytical skills helped you manage risk in a project or decision?
Analytical skills have helped me manage risk by enabling me to assess and quantify uncertainties, identify potential risks, and develop risk mitigation strategies. By conducting risk analysis, scenario modeling, and sensitivity testing, I can anticipate potential outcomes, evaluate their impact on project objectives, and prioritize risk mitigation actions. Analytical skills also facilitate data-driven decision-making, allowing me to evaluate trade-offs, optimize resource allocation, and implement contingency plans to manage risks effectively and ensure project success.
22. Can you give an example of how you've used data visualization to support your analytical findings?
Certainly. In a project analyzing sales performance across regions, I used data visualization tools such as charts, graphs, and heat maps to present key insights and trends effectively. By visualizing sales data geographically, I identified regional sales patterns, market opportunities, and areas for improvement. I created interactive dashboards that allowed stakeholders to explore data dynamically, enabling them to gain actionable insights and make informed decisions based on visualized trends and performance metrics. Data visualization enhanced communication, facilitated understanding, and drove alignment among stakeholders, supporting the implementation of targeted strategies and initiatives to drive business growth.
23. How do you approach learning and applying new analytical methodologies?
I approach learning and applying new analytical methodologies by following a structured process. First, I identify the specific methodology or tool I want to learn based on its relevance to current projects or industry trends. Then, I engage in self-paced learning through online courses, tutorials, and reading relevant literature to understand the underlying principles and techniques. I practice applying the methodology to real-world datasets or simulation exercises to gain hands-on experience and reinforce learning. I seek feedback from peers or mentors, participate in collaborative projects, and attend workshops or webinars to exchange knowledge and best practices. I also stay updated on advancements in analytical methodologies by following industry publications, attending conferences, and exploring emerging tools or technologies, ensuring continuous growth and adaptation in my analytical skill set.
24. What is the most challenging analytical problem you've faced, and how did you solve it?
The most challenging analytical problem I faced was in developing a predictive model for fraud detection in financial transactions. The complexity arose from the dynamic nature of fraud patterns, evolving tactics used by fraudsters, and the need for real-time detection to minimize losses. To address this, I collaborated with data scientists and domain experts to gather extensive historical data on fraudulent transactions, customer behaviors, and risk indicators. I used advanced machine learning algorithms such as anomaly detection, clustering, and ensemble methods to identify fraudulent patterns and improve model accuracy. I also implemented automated alerts and risk scoring mechanisms to flag suspicious activities in real time, enabling proactive intervention and reducing fraud losses significantly.
25. How do you ensure that your analytical models are both effective and efficient?
I ensure that my analytical models are effective and efficient by following best practices in model development, validation, and optimization. I start by defining clear objectives, selecting appropriate algorithms, and preprocessing data to improve model performance. I split data into training, validation, and test sets to assess model accuracy, generalization, and robustness. I use techniques like cross-validation, hyperparameter tuning, and feature selection to optimize model performance and avoid overfitting. I also conduct model performance monitoring, retraining, and recalibration to adapt to changing data patterns and ensure ongoing effectiveness and efficiency of the models.
26. Can you explain how you've used statistical methods to inform your analysis?
Statistical methods play a crucial role in informing my analysis by providing quantitative insights, hypothesis testing, and validation of findings. For instance, I use descriptive statistics to summarize and visualize data distributions, central tendencies, and variability. I apply inferential statistics to make inferences and draw conclusions about populations based on sample data, using techniques like hypothesis testing, confidence intervals, and regression analysis. I also use multivariate statistical methods such as factor analysis, cluster analysis, and regression modeling to uncover patterns, relationships, and predictive insights in complex datasets. Statistical methods help me validate assumptions, quantify uncertainties, and make evidence-based decisions, enhancing the rigor and reliability of my analytical work.
27. How do you handle feedback or criticism of your analytical conclusions?
I handle feedback or criticism of my analytical conclusions by adopting a constructive and open-minded approach. I welcome feedback as an opportunity for learning, improvement, and refinement of my analyses. I listen actively to understand perspectives, ask clarifying questions, and seek additional context or data to address concerns. I engage in collaborative discussions, present supporting evidence or rationale for my conclusions, and consider alternative interpretations or viewpoints. I take ownership of any mistakes or limitations in my analysis, acknowledge feedback graciously, and use it to iterate and enhance the quality and credibility of my analytical work.
28. What strategies do you use to ensure your analytical work remains objective and unbiased?
To ensure objectivity and minimize bias in my analytical work, I follow several strategies. First, I define clear research questions or objectives upfront to guide my analysis and avoid confirmation bias. I use random sampling, stratification, or other sampling techniques to reduce selection bias and ensure representative data samples. I apply robust statistical methods, control variables, and conduct sensitivity analyses to account for potential biases or confounding factors. I document my methodologies, assumptions, and decision-making processes transparently to facilitate scrutiny and validation by peers or stakeholders. I also seek diverse perspectives, encourage constructive criticism, and maintain a critical mindset to challenge assumptions, validate conclusions, and promote objectivity and rigor in my analytical work.
29. Can you describe a time when you had to use analytical skills to improve a team or organizational process?
Certainly. In a previous role, I used analytical skills to improve inventory management processes for a manufacturing team. I conducted a comprehensive analysis of inventory levels, production schedules, lead times, and demand forecasts. Using statistical modeling and optimization techniques, I identified opportunities to streamline procurement processes, reduce excess inventory, and minimize stockouts. I collaborated with cross-functional teams to implement automated inventory tracking systems, reorder point algorithms, and supply chain optimization strategies. This resulted in a 20% reduction in inventory holding costs, improved production efficiency, and enhanced customer satisfaction due to more reliable product availability, demonstrating the impact of analytical skills in optimizing team and organizational processes.
30. How do you assess the impact of your analytical work on business outcomes?
I assess the impact of my analytical work on business outcomes by defining key performance indicators (KPIs), setting measurable goals, and establishing benchmarks or baseline metrics to track progress. I conduct pre- and post-analysis comparisons to evaluate the effectiveness and ROI of analytical initiatives. I use quantitative metrics such as revenue growth, cost savings, customer retention rates, or operational efficiency improvements to quantify the impact on business outcomes. I also solicit feedback from stakeholders, conduct surveys or interviews, and analyze qualitative data to assess the perceived value, usability, and relevance of analytical insights in driving decision-making and achieving strategic objectives. Regular performance reviews, continuous monitoring, and ongoing optimization efforts help me ensure that analytical work aligns with business priorities, adds value, and contributes to positive outcomes for the organization.
31. In what ways have you used technology to enhance your analytical capabilities?
I have leveraged technology extensively to enhance my analytical capabilities. For data processing and manipulation, I use tools like Python and R programming languages, along with libraries like Pandas and NumPy, which enable efficient data handling and manipulation. For data visualization, I utilize tools such as Tableau and Power BI to create interactive dashboards and visually communicate insights effectively. Machine learning frameworks like scikit-learn and TensorFlow have been instrumental in developing predictive models and advanced analytics. Additionally, I stay updated with emerging technologies and trends in data analytics, cloud computing, and big data platforms, which further enhance my analytical toolkit and enable me to tackle complex analytical challenges more effectively.
32. How do you manage time effectively when conducting complex analyses?
To manage time effectively during complex analyses, I employ several strategies. I start by breaking down the analysis into manageable tasks and setting clear milestones or deadlines for each phase. I prioritize tasks based on urgency, importance, and dependencies, focusing on high-impact areas first. I use project management tools like Jira or Trello to track progress, allocate resources, and collaborate with team members efficiently. I also practice time blocking, dedicating uninterrupted blocks of time for deep analysis and concentration. Regular checkpoints, progress reviews, and agile methodologies help me stay on track, adapt to changes, and deliver quality results within timelines.
33. Can you provide an example of a situation where you had to teach or mentor someone in analytical techniques?
Certainly. I had the opportunity to mentor a junior analyst in my team who was new to machine learning techniques. I structured a mentoring program that included hands-on workshops, code reviews, and collaborative projects to enhance their understanding and practical application of analytical techniques. I provided personalized guidance, resources, and feedback to help them learn Python programming, data preprocessing, feature engineering, and model building. Through pair programming sessions and regular knowledge-sharing meetings, I facilitated their transition from basic analytics to advanced machine learning concepts. As a result, the mentee gained confidence, improved their analytical skills, and successfully contributed to project outcomes, demonstrating the value of mentorship in developing analytical talent within the team.
34. What ethical considerations do you take into account when performing an analysis?
Ethical considerations are paramount in performing analysis, and I adhere to ethical guidelines and principles throughout the process. I prioritize data privacy and confidentiality, ensuring compliance with regulations such as GDPR or HIPAA and obtaining necessary permissions for data usage. I maintain transparency and integrity by documenting data sources, methodologies, and assumptions transparently. I avoid bias and ensure fairness by using representative samples, unbiased algorithms, and considering diverse perspectives in analysis. I respect intellectual property rights, avoid plagiarism, and cite sources appropriately. Additionally, I communicate findings responsibly, avoid misleading interpretations, and consider potential impacts on stakeholders and society, aiming for ethical and socially responsible outcomes in my analytical work.
35. How do you approach troubleshooting when your analysis does not proceed as expected?
When my analysis does not proceed as expected, I adopt a systematic troubleshooting approach. I review data quality and preprocessing steps to identify any issues or anomalies in the data. I check for errors in coding, algorithm implementation, or model assumptions that may affect results. I conduct sensitivity analyses, robustness checks, and diagnostic tests to understand variations and inconsistencies in outcomes. I collaborate with domain experts, data scientists, or peers to brainstorm ideas, validate assumptions, and explore alternative methodologies or approaches. I document troubleshooting steps, lessons learned, and revised analyses transparently to facilitate learning, continuous improvement, and reproducibility in future analyses.
36. Can you discuss how you use analytical skills to contribute to strategic planning?
Analytical skills are instrumental in contributing to strategic planning by providing data-driven insights, informed decision-making, and actionable recommendations. I start by analyzing historical data, market trends, competitive landscapes, and internal performance metrics to identify strengths, weaknesses, opportunities, and threats (SWOT analysis). I use scenario analysis, forecasting models, and predictive analytics to anticipate future scenarios, assess potential risks, and evaluate strategic options. I conduct market segmentation, customer profiling, and demand forecasting to inform product development, pricing strategies, and market entry decisions. I collaborate with cross-functional teams, senior leadership, and external stakeholders to align objectives, set strategic priorities, and develop implementation plans that leverage data-driven insights for sustainable growth and competitive advantage.
37. How do you balance detailed analysis with the need to meet deadlines?
Balancing detailed analysis with meeting deadlines requires effective time management, prioritization, and strategic allocation of resources. I start by defining project scopes, objectives, and key deliverables upfront to align with stakeholder expectations and timeline constraints. I break down the analysis into manageable tasks, set milestones, and allocate time based on the complexity and criticality of each task. I use agile methodologies, iterative approaches, and regular progress reviews to track milestones, identify bottlenecks, and adapt plans as needed. I prioritize high-impact analyses and focus on key insights that drive decision-making, while also ensuring that detailed analyses are conducted efficiently without compromising quality. I communicate proactively with stakeholders, manage expectations, and negotiate realistic timelines when necessary to ensure a balance between thorough analysis and meeting deadlines effectively.
38. In what ways have you contributed to improving analytical practices within your team or organization?
I have contributed to improving analytical practices within my team and organization in several ways. I championed the adoption of best practices and standards in data management, analysis, and reporting to ensure consistency, accuracy, and reproducibility in analytical workflows. I led training sessions, workshops, and knowledge-sharing initiatives to upskill team members in advanced analytical techniques, tools, and methodologies. I promoted a culture of data-driven decision-making by establishing data governance frameworks, quality assurance processes, and performance metrics to monitor and optimize analytical outcomes. I collaborated with IT and data engineering teams to streamline data integration, automate repetitive tasks, and enhance data accessibility for analytical purposes. I also encouraged innovation, experimentation, and continuous improvement in analytical approaches, fostering a collaborative and learning-oriented environment that drives excellence and value creation through analytics across the organization.
39. How do you determine which analytical approach is most appropriate for a given problem?
Determining the most appropriate analytical approach for a problem involves a thorough understanding of the problem's nature, data availability, desired outcomes, and constraints. I start by clarifying the objectives and defining key metrics that align with business goals. I assess the type of data (structured or unstructured), its volume, quality, and relevance to the problem. Based on these factors, I evaluate different analytical approaches such as descriptive analytics for insights generation, diagnostic analytics for root cause analysis, predictive analytics for forecasting, or prescriptive analytics for decision optimization. I consider the complexity of the problem, time constraints, and resource availability to select the approach that balances accuracy, feasibility, and actionable insights. Consulting with domain experts, stakeholders, and leveraging past experiences also guides me in choosing the most appropriate analytical approach for effective problem-solving.
40. Can you share an experience where you used cross-functional knowledge to enhance your analysis?
Certainly. In a cross-functional project, I collaborated with marketing, sales, and finance teams to analyze customer churn and identify retention strategies for a subscription-based service. My background in data analytics, combined with insights from marketing campaigns, sales performance data, and financial metrics, allowed me to conduct a comprehensive analysis. I integrated customer behavior data, demographic information, and transaction histories to segment customers, identify churn patterns, and predict potential churn risks using machine learning models. By leveraging cross-functional knowledge and diverse perspectives, we developed targeted retention initiatives, personalized offers, and customer engagement strategies that led to a significant reduction in churn rates and increased customer loyalty, highlighting the value of cross-functional collaboration in enhancing analytical outcomes.
41. How do you ensure that your analytical findings are actionable?
Ensuring that analytical findings are actionable involves several key steps. First, I frame the analysis around specific business objectives and key performance indicators (KPIs) that align with actionable outcomes. I involve stakeholders early in the process to understand their requirements, priorities, and decision-making needs. I present findings in a clear, concise, and actionable format using data visualizations, executive summaries, and actionable recommendations. I quantify the impact of recommendations, prioritize actionable insights based on potential ROI or strategic importance, and provide implementation guidance, timelines, and success metrics. I facilitate discussions, address questions or concerns, and collaborate with stakeholders to develop action plans, allocate resources, and track progress towards achieving desired outcomes. Regular follow-ups, performance monitoring, and feedback loops ensure that analytical findings translate into tangible actions and measurable results for the organization.
42. What is your experience with predictive analytics?
My experience with predictive analytics includes a range of applications across industries. I have developed predictive models for customer segmentation, churn prediction, demand forecasting, risk assessment, and recommendation systems. I have used regression analysis, decision trees, random forests, neural networks, and ensemble methods to build predictive models that leverage historical data, patterns, and trends to make future predictions and recommendations. I have worked with large datasets, data preprocessing techniques, feature engineering, and model evaluation methods to optimize predictive accuracy, interpret model outputs, and validate model performance. I have also implemented predictive analytics solutions in real-time environments, integrated models into business processes, and measured the impact of predictions on business outcomes, demonstrating the value of predictive analytics in driving data-driven decision-making and strategic planning.
43. How do you maintain your focus and attention to detail when performing repetitive analytical tasks?
Maintaining focus and attention to detail during repetitive analytical tasks requires discipline, organization, and effective time management strategies. I start by breaking down tasks into smaller subtasks or workflows to maintain clarity and structure. I create checklists, templates, and standardized procedures to ensure consistency and reduce errors. I use productivity techniques such as the Pomodoro Technique, time blocking, and regular breaks to manage focus and avoid burnout. I leverage automation tools, scripts, and macros to streamline repetitive tasks, minimize manual intervention, and improve efficiency. I also periodically review and validate outputs, perform quality checks, and seek feedback from peers or supervisors to ensure accuracy and reliability in my work. Continuous learning, skill development, and goal setting help me stay motivated, engaged, and committed to delivering high-quality results consistently in repetitive analytical tasks.
44. Can you discuss a time when you had to use analytical skills to negotiate or influence a decision?
Certainly. In a strategic planning project, I used analytical skills to influence a decision regarding resource allocation and investment priorities. I conducted a comprehensive analysis of market trends, competitive landscapes, customer preferences, and financial projections to evaluate different growth opportunities and strategic initiatives. I developed scenario analyses, sensitivity models, and business cases to quantify potential risks, returns, and strategic implications of each option. I presented data-driven insights, risk assessments, and ROI estimates to senior leadership and key stakeholders, highlighting the strategic alignment, value proposition, and impact of recommended initiatives. Through persuasive communication, compelling storytelling, and evidence-based arguments, I influenced decision-makers to prioritize high-impact projects, reallocate resources effectively, and align investments with long-term business goals, showcasing the power of analytical skills in driving informed decisions and strategic outcomes.
45. How do you deal with conflicting data or opinions when conducting an analysis?
Dealing with conflicting data or opinions during analysis requires a diplomatic, collaborative, and evidence-based approach. I start by seeking clarity on data discrepancies, sources of bias, or conflicting interpretations through open communication and active listening. I engage stakeholders, subject matter experts, and data owners to validate data integrity, resolve discrepancies, and reconcile differences in opinions or assumptions. I conduct sensitivity analyses, robustness checks, and alternative scenarios to assess the impact of conflicting data on analysis outcomes and decision-making. I facilitate discussions, encourage diverse perspectives, and mediate constructive debates to reach consensus, clarify misunderstandings, and align on common goals. I document discussions, decisions, and rationales transparently to foster accountability, traceability, and continuous improvement in analysis processes. By promoting collaboration, transparency, and data-driven decision-making, I navigate conflicting data or opinions effectively, ensuring analytical rigor and credibility in my work.
46. What role do you think analytical skills will play in the future of your industry?
I believe that analytical skills will play a pivotal role in shaping the future of my industry by driving innovation, competitive advantage, and informed decision-making. With the proliferation of data sources, digital technologies, and advanced analytics capabilities, organizations across sectors are increasingly leveraging data-driven insights to gain a deeper understanding of market dynamics, customer behaviors, and operational performance. Analytical skills such as data mining, predictive modeling, machine learning, and data visualization will continue to be in high demand to extract actionable insights, uncover hidden patterns, and optimize business processes. The ability to translate data into strategic initiatives, identify growth opportunities, and mitigate risks will be critical for staying competitive and achieving sustainable growth in a data-driven economy. Moreover, ethical considerations, data governance, and responsible use of data will become essential aspects of analytical skills, ensuring trust, transparency, and value creation for stakeholders and society as a whole. As the pace of technological advancements accelerates, continuous learning, adaptability, and interdisciplinary collaboration will be key enablers for professionals with strong analytical skills to thrive and drive positive impact in the future of my industry.
47. How do you incorporate feedback into your analytical process?
Incorporating feedback into the analytical process is essential for refining insights, validating assumptions, and improving decision-making. I start by actively seeking feedback from stakeholders, domain experts, and end-users throughout the analysis lifecycle. I gather feedback on data quality, relevance of analysis objectives, model assumptions, and actionable insights. I use feedback mechanisms such as surveys, interviews, focus groups, and user testing to understand perspectives, gather qualitative insights, and identify areas for improvement. I integrate feedback iteratively into data collection, preprocessing, model development, and interpretation stages to validate findings, address concerns, and enhance the robustness and relevance of analytical outcomes. I document feedback, actions taken, and outcomes transparently to promote accountability, continuous learning, and stakeholder engagement in the analytical process, ensuring that insights generated align with stakeholder needs and drive meaningful impact.
48. Can you explain how you've used analysis to improve customer satisfaction or user experience?
Analysis has been instrumental in improving customer satisfaction and user experience through data-driven insights, personalized recommendations, and targeted interventions. For example, in a customer feedback analysis project, I analyzed survey responses, sentiment data, and interaction patterns to identify pain points, preferences, and opportunities for enhancement in a mobile application. I conducted text analytics, sentiment analysis, and clustering techniques to categorize feedback, prioritize issues, and uncover underlying themes affecting user satisfaction. Based on analysis findings, I collaborated with UX designers and product teams to redesign user interfaces, streamline workflows, and introduce new features that address customer needs and preferences. I monitored key metrics, conducted A/B testing, and measured the impact of changes on user engagement, retention, and satisfaction scores. The iterative analysis-feedback-improvement cycle led to measurable improvements in user experience, higher customer satisfaction ratings, and increased app adoption, showcasing the value of analysis in driving continuous improvement and customer-centricity.
49. What is the most innovative analytical technique you've applied in your work?
One of the most innovative analytical techniques I've applied is machine learning anomaly detection for fraud detection in financial transactions. I used unsupervised learning algorithms such as Isolation Forest and Local Outlier Factor (LOF) to identify unusual patterns, outliers, and potential fraud instances in large-scale transactional data. By leveraging feature engineering, dimensionality reduction, and model tuning techniques, I developed a robust anomaly detection system that effectively flagged suspicious transactions, unusual spending behavior, and fraudulent activities in real-time. The system integrated with existing fraud prevention mechanisms, alerting mechanisms, and case management workflows to enable prompt investigation, mitigation, and prevention of fraudulent activities. The innovative use of machine learning for anomaly detection not only improved fraud detection accuracy but also reduced false positives, operational costs, and risks associated with financial fraud, demonstrating the power of advanced analytical techniques in addressing complex business challenges.
50. How do you balance the need for thorough analysis with the urgency of making timely decisions?
Balancing thorough analysis with the urgency of making timely decisions requires a strategic approach, effective prioritization, and agile decision-making processes. I start by understanding the criticality and impact of decisions on business objectives, risk tolerance, and stakeholder expectations. For time-sensitive decisions, I focus on key variables, critical assumptions, and high-impact factors that drive outcomes, prioritizing depth of analysis based on decision urgency and complexity. I use rapid prototyping, iterative modeling, and decision trees to simulate scenarios, assess trade-offs, and identify decision thresholds that guide action. I leverage pre-built analytics templates, automated workflows, and decision support tools to expedite data preparation, model deployment, and insights generation. Collaborating with cross-functional teams, subject matter experts, and decision-makers in agile frameworks enables quick feedback loops, adaptive responses, and collaborative decision-making that balance analytical rigor with decision timeliness. I also establish escalation protocols, decision criteria, and contingency plans to address uncertainties, minimize risks, and ensure that timely decisions are data-informed, evidence-based, and aligned with strategic objectives, fostering a culture of agility, resilience, and informed decision-making in dynamic business environments.